Burç Kostem pointed me me to this wonderful piece by Matteo Pasquinelli on the history of neural networks. In the middle, there’s a small historical detail that I never quite grasped before:
In 1969 Marvin Minsky and Seymour Papert’s book, titled Perceptrons, attacked Rosenblatt’s neural network model by wrongly claiming that a Perceptron (although a simple single-layer one) could not learn the XOR function and solve classifications in higher dimensions. This recalcitrant book had a devastating impact, also because of Rosenblatt’s premature death in 1971, and blocked funds to neural network research for decades. What is termed as the first ‘winter of Artificial Intelligence’ would be better described as the ‘winter of neural networks,’ which lasted until 1986 when the two volumes Parallel Distributed Processing clarified that (multilayer) Perceptrons can actually learn complex logic functions.
In terms of the emerging historiography of machine learning, this is a place where the whig historians (aka, internalist histories by computer scientists) and the critical historians seem to agree.
But XOR as a test of neural networks, or intelligence, is a very interesting reduction of pattern recognition to a binary option. It is basically a binary calculation that compares two inputs. It answers “yes” if the two inputs are the different, and “no” if the two inputs are the same. Here’s a picture from Wikipedia to illustrate:
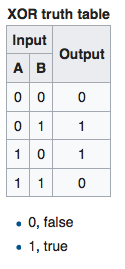
The whole political argument around pattern recognition in AI is basically reducible to what counts as a 1 and what counts as a 0 in that B column: this is why Google had to block its image recognition algorithms from identifying gorillas. Solving for XOR (are they different) or XAND (are they the same?) is never quite enough in real cultural contexts.
Here’s why. Let’s consider this against a famous statement of morphological resemblance from the history of social thought:
Is it surprising that prisons resemble factories, schools, barracks, hospitals, which all resemble prisons?
(Michel Foucault, Disipline and Punish)
This is an argument about general morphology. It is not that prisons and schools are exactly the same but that they share some meaningful aspects. Can an AI be trained to understand whether or not a given social arrangement fits a “panoptic diagram”? Only if you can parameterize every element of the description of a social milieu.
So we now have a media environment where neural nets can and do solve for binary logic functions all the time. The question is what trips that switch from 0 to 1 in either column. It is, in other words, built around a politics of classification.
For all the talk about process fairness and ethics in AI, we know from the history, anthropology, and STS study of classification that classifications are always tied to power.